Dear VC’s, please stop throwing money at AI founders with no commercial plan, besides AGI
Execute a commercial plan from day 0, not next year in the future, when the bank is empty
A wave of AI foundation model companies, with half a billion dollars raised - reaching their end
Throughout 2023, we see headline after headline. Of various all-star teams, pulling researchers from the top of FAANG, Ivy leagues, Open AI. To create their Avengers of AI.
Since 2024, we have seen the fall, one after another, after asking 100’s of millions after 100’s of millions. Reach their end, as they run out of money
But as far as I am concerned - what weed were you VC’s and Founders smoking?
Everyone was trying to follow the same blueprint, of openAI oversimplified
Step 1: Get the best researchers, Spend $XX million a month, researching and building the best model out there
Step 2: Keep researching, get results, scale up, Ask for more money for each milestone
Step 3: ???
Step 4: Profit; and make a billion-dollar business
The AI market is no longer the same as it was pre-OpenAI - the formula was never reliably repeatable in the first place.
AI does not remove the fundamental principle of businesses needing to make money
But, but … we funded the team that had all the brightest AI researchers, from Google, openAI, Ivy leagues …
That's exactly part of the problem, of how VC’s oversimplified the whole thing. As much as I love their work, what do you think researchers will do with funding…. Research!
Do you not know what the life cycle for a researcher is?
Step 1: Propose a research idea, get funding grant approval
Step 2: Get results, Write papers, and Scale up. Ask for more money for each milestone
Step 3: Repeat steps 1 & 2, with more ideas, or bigger ideas
Step 4: Accumulate enough paper citations, get university tenure, retire
Noticed how in the researcher's life cycle, at no point was it about monetizing, making profits, or making a billion-dollar business. If anything, research by its nature is very capital-inefficient.
While they are undoubtedly great brilliant minds in research (and I love them for their contributions). They are not the right people to turn research into commercial products. And inversely, ask for more and more money.
While leaving the commercialization process to “someone else”.
VC’s correlated PhDs & papers to commercial success. Over-indexing to OpenAI's outward appearance of success. When literally, some of the most profitable, and capital-efficient teams in AI have the inverse correlation
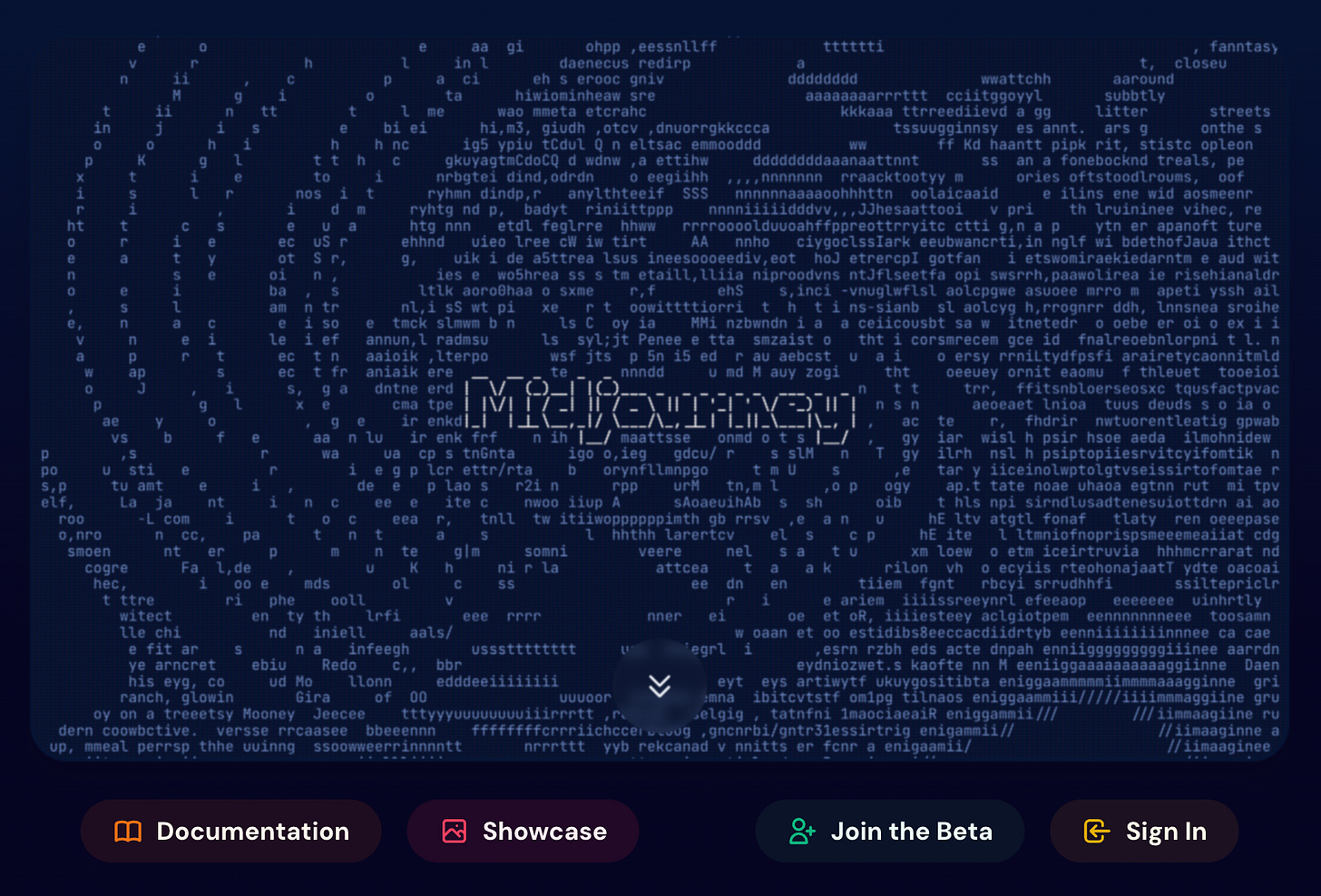
Wrong Conclusion: OSS / Foundation AI is too expensive?
The continuous repeat of the extremely large funding cycle of 2023, with larger and larger funding cycles, from foundation model companies. Have lead to extremely wrong conclusions from the VC markets
That foundation model companies require half a billion dollars and more to succeed
Open-source AI companies can't succeed.
This could not be further from the truth. GPT-4 is estimated to have a training cost of $100 Million in GPU time. And with advancements in AI techniques and technology, this cost has been going down (not up).
Add in labor, and various costs - Any company that raised beyond $250 Million and failed to catch up with GPT-4 is just being “capital inefficient”. A number that is well below many foundation model 2023 capital raise.
So why do they keep failing? It’s back to the basics of “lack of focus” and “financial discipline”. And distracted by research
This can be best reflected by, a failure of the following basic test ….
Litmus Test: “Time to pricing, and payment”
In the same naive mistake, that countless Open Source Companies have encountered, foundation model companies went on the same blind trend of “figuring out how to make money too late”.
And I say this, not only as an observer but as a passionate User as well …
I love you all, but ….
In Rancher's case: I have given talks, workshops, and evangelized ranchers into other startups. But have contributed $0.
I use their product daily (self-hosted), and would pay to have a good managed SaaS solution, which never existed.
The only current way to pay them is to be a giant enterprise with a crazy expensive custom enterprise contract, which I am not.
In Stability AI case: I love you, folks. As a GPU donor recipient for the RWKV OSS projects (thanks!). I was literally trying to help pay you, in some shape and form, to help improve your numbers for your investors. But gosh do you make it impossible! I had to fight your system to give you money.
The paid image generation platform (dreamstudio), is nowhere to be found on your home page and is constantly on page 3 of Google for text-to-image. Refuses a subscription, and is only credits-based. I have to bookmark it, so I can use it every now and then when I need an AI image generated. To effectively give it a few dollars every now and then.
The model membership, for $20/month, requires me to fill up a form that requires manual review - only to have the reviewer inform me that I do not qualify. As I was not having enough users & making enough money. In both cases I know this, I just want to give you $20/month
For Inflection AI case: How does anyone even pay you, there is no enterprise sales form, nor cloud sales process on your page.
There are already enough problems getting pricing right, for any SaaS/platform growth …
But to make it impossible for customers who want to give you money, to give you any. Is in my opinion the complete opposite of “financial discipline”, which is so self-damaging, it’s investor harm.
Unless your plan was a pure acquisition exit. If you want to make a stable company, constantly releasing wonderful open source code or AI models to the public. You need to have an income stream. Employees need to be paid, Servers / GPUs need to be paid. Investors need to be paid an ROI.
As a business, your number one primary goal is to make Money. We can discuss common good, altruism, etc - AFTER - we make sure your business, is stable enough to stay around and pay the bills while making money in excess.
Even charities and non-profits need a plan on how to get donation money. So does your business, noble goals, and idealistic open source visions aside.
This is a problem not just for AI, but any large-scale open-source project in general. A problem we have seen repeated many times. With idealistic views.
We can do better ….
On the other spectrum, is established companies with Open Source/Core models: MongoDB and Elastic. WIth Open Source/Core products that I have gotten either my startup or other startups I advise to be paying for.
The time to pricing, and payment is relatively straightforward within a few clicks.
It's so straightforward, that this revenue funnel, from one-click users to enterprises - makes them a revenue of $1.5 Billion / $1.1 Billion dollars per year respectively, with an R&D budget of over $300M per year for both.
Enough revenue, or R&D budget, to be training GPT-4 multiple times a year. Enough R&D spend, to outspend the 3 other companies I highlighted, that failed this basic litmus test.
This is despite, having everything about their Open stack being potentially an investor nightmare
Their software stack has been cloned by every major cloud provider and competitor
Their open source / open core offering is complete enough, that yes - I can actually self-host everything if I want to - and I do so for my own personal cluster usage
But why do I and several others, then decide to pay them, instead of their cloned offerings - to a tune of billions of dollars
Production Reliability: Scaling up AI and Databases safely and reliably is incredibly hard, especially in production where you cannot afford any downtime.
Convenience in scaling up and down: Related to production reliability
Convenience from the Source: Ease of upgrade and access to updates, before any other providers
Reasonable Pricing: Without crazy per-seat pricing, it's not much cheaper to get the same performance/service offering on your own, once you factor in upgrade processes required, hardware redundancy, etc. Both Elastic, and MongoDB inversely, get to leverage their economics at scale, and benefits as part of their margins.
Multi-cloud offering: allow me to easily move between AWS, GCP, and Azure if needed
Enterprise compliance and security (ISO, and SOC certification processes)
Essentially make it more attractive for me to use your solution and hardware. Then my own cloud hardware, and we the user will pay.
Wrong Conclusion: Is the pricing page all I need?
It is a start and is infinitely better than being user-hostile in making money.
It may be sufficient to get to the thousands, or maybe millions in revenue. But if you want to reach billions, it's all about the details …
Moving from research to production, and sales is a muscle!
A muscle that rarely any one company gets right the first time round, a muscle that needs to be constantly trained and iterated.
Notice the list of reasons previously, on why I and many others choose to pay for the commercial offering. Despite even having the expertise to self-host on our own?
Without exercising the muscles from converting a research product into production and sales, it is easy to make the following mistakes
Poor production reliability: Rate limits, Downtime, all constantly puts off users
Missing features that the customer wants: from paid offerings, which the open source offerings provide
Poor pricing: Users are not idiots, we will shop around, and get pricing drastically wrong compared to your peers. And we will switch. Inversely, price too low, and the company gets burnt.
Missing conveniences: A better UX/DX, is a very large reason why your commercial offering will be preferred over DIY.
Bad sales processes: Your enterprise sales team, needs time to find Product Market Fit, needs time to scale.
While I can sing the praises of Mongo, elastic, or even supabase (Postgres). I can also point out various open-source technology projects, which failed on some version of these points badly enough. That their users were pushed into self-hosting or other solutions.
There is no one-size-fits-all solution to these issues, there is only going to market, and iterating with the users. Getting this right for B2B / developers and enterprises is complicated enough entire investors and courses is specializing in this segment (eg. heavybit)
We know all of these things, We have learned it through the cycles of various deep tech startups, and B2B SaaS. Sure investors may have been blinded by greed to repeat OpenAI, through oversimplified pattern matching ( Big name + AI = Profit ?? ).
But why do founders, even business founders, avoid building those muscles - when they know better ???
The problem of incentives from VC’s …
Foundation model / AI companies by their very nature are deep tech. It requires a fairly deep capital of 50M and up.
On the flip side, consumers are very demanding, and besides notable exceptions, are mostly only willing to consider paying once a model reaches GPT 3.5 class and up approximately.
1) VC’s Overfitting to the Product Market Fit revenue rocket
One of the notable issues, that founders constantly discuss - is that once you have revenue - VC’s tend to only focus on the revenue and Product Market Fit curve. Which boils down to: Is it a rocket or not?
This creates a weird situation, where strategically speaking we founders are aware of the uphill sales cycle for anything below a GPT 3.5 class model.
As such, It makes more sense to avoid any revenue until you have an AI model approaching the GPT 3.5 class, after which you can start building a platform. This process is something even several VCs will advise founders on, to maintain focus …
Knowing the tall task involved, many opted to focus on benchmark and open source adoption first before any commercialization … but that has its own weaknesses …
2) Worry about the revenue later instead? You are going to get sniped.
This is what happened to Mistral, as they scaled their model up to Mixtral …
Only to find competing providers, to not only enter the market first to the public before them. But to do so at a lower price point. With their model.
All of which was to be partially expected - because, while Mistral was busy entirely just creating the model - they never built that muscle for bringing their very own model into production and optimizing the user convenience to the sales process. They were isolated from the users.
Inversely, together AI has already been, running the Mistral 7B model. Iterating with the users, on every factor, from convenience to price. It may not have been a major success, but they have gotten the iteration process going. As such, when the mixtral model came out, they were more prepared than the mistral team to publish their API for the model.
Together AI has been exercising the production shipping, and commercial muscle. Mistral didn’t
3) Making it worse: build it close source, behind the scenes? Scale up when we are ready
Basically what Inflection, and a few others did.
If they avoided revenue, they were forced to be working on the model, without user feedback …
If they accepted revenue, they will face an uphill task of making consumers care … because they were neither GPT 3.5 class nor open source which they can tinker with … Additionally investors would be doubting them in the early stages due to 1)
This is also why - nearly every AI startup is a “beta” in the early stages, to have some form of customer users and feedback loop. Without committing to the revenue hockey stick.
Basically, you escalate the stakes. You either clearly beat and provide a strong reason to move from the existing close source model with a Unique Selling Point. With very limited user feedback, till you get to that point.
And if you fail, you will have ended up creating a model, which no one wanted (as it was not adapted to user feedback). A model and platform that no one cared about (as it is not open source), with little to no revenue nor user adoption.
Sounds hard right? Well there is an answer … An alternative …
How Should it be done then? How does one build a foundation model company?
You need a founding CEO
who has both enough domain expertise and respect in AI to lead an AI team
with business sensibility,
to actually steer the company to profit
and not burn more than 100~200 M to make GPT4 class AI
Why not a business CEO, with a AI researcher CTO?
You will need them both to be very very aligned. Hard decisions will need to be made that involves a quarter to half of the company capital. A decision which can only be made with informed context of both the AI training process and business goals. Decisions which either side may fear making or misjudge, due to lack of context of the other side.
With a founding CEO / CTO / Team, that has some mix of the following
dataset and AI model training and scaling experience
who has experience building and scaling resource-hungry infrastructure platforms (for inference)
sales and marketing muscles with either (or both)
a DevRel team to help promote the platform adoption
or an Enterprise sales and partnership team to close the deals
What if there are talents lacking in specific segments?
That is fine, its hard enough to find the combination of talent required for building and scaling AI models (There are less then 1000 individuals who has experience training a 7B and above model from scratch). Let alone everything else. Discuss with the founding team, a recruitment plan for the required gaps in the team.
With a plan to scale in steps, both the model and platform
Stage 1: Something to prove the concept / technical approach
Stage 2: Initial platform and model, which can start closing some customers (even if it is ineffective, unscalable, or slow)
This can ideally be within a specific market niche or use case
Helps ensure all initial scaling issues on the platform are tackled, and sorted out
Stage 3: Scaled up, highly performant model and platform, tuned heavily to the user needs (where the hockey stick begins)
Ramping up on sales and customer adoption
Maybe split into smaller sub-stages (eg. GPT 3.5 class, GPT 4 class)
All in under 2 years, because AI moves fast
With Investors, who are willing to commit to such a plan, step by step.
Can’t we skip stage 1 & 2, and go straight to 3?
Unless you are able to find the required talents who have done it all before. Which you do not, as they are all concentrated within OpenAI and Anthropic (not even google counts). Across all the various key disciplines. Then maybe you can remove stage 1 & 2?And even then, that would be a high risk move to an already high risk endeavor. Its better to just sort out the bumps, across a few months, and the customer feedback loop. To get this right.
For context, openAI had the time between 2018-2020 for stage 1 (GPT-1 & 2). Followed by 2 years+ of stage 2, with early user traction and iteration (GPT-3) between 2020-2022 And only stage 3, towards the end of 2022, early 2023.
But yea, many VC’s loved to ignore the first few stages, and only focus on replicating the last stretch. Without realizing how hard and essential it is to make sure to sort out the platform at scale. After all this is not just a “SaaS template” + SuperBase combo. The success and failure is in the details.
And dun forget to make a profit?
By selling the AI product, at a price point that - actually makes money this time!
OpenAI is reported to be making losses of near $500M a year, or over a million dollar a day. According to various reports, including buisnessinsiders.com
The last part of making profits, making money, over the cost of goods - is important because
You can cover all bills, to be default alive
You can funnel the revenue to future models, and customer growth, To grow future revenue
And recursively scale up the business, and AI model in the process.
Because why not? The market is hungry, over a 100 billion dollars a year hungry, if you get the pricing and scaling right even with existing AI models (GPT 3.5 and GPT 4 class)
Sounds like a saner business plan - better than trying to raise 7 Trillion dollars right?
A self-fullfilling loop that keep scaling past even AGI?
Well, do we have something for you …
Guess what! - that's basically what, me and my team are doing here are Recursal AI are doing.
We are built using a new AI architecture: That can make a profit, as it’s 100x++ cheaper on inference compared to existing AI architecture (transformers).
We plan to make not just revenue, but profits: Its part of our founding story, we grew from the ashes of un-stable AI companies. And never want that to happen again
We do not plan to raise more than $250M: Because, we have always been capital-efficient, and plan to stay that way.
We just finished Stage 1 (while spending <$2.5M):
We proved our architecture, can be scaled like transformers and even outperform LLaMA 2 7B
Multiple orders of magnitude more capital efficient then any LLM group
We are now at Stage 2:
We have our initial commercial cloud platform, and strategic partnerships (more details to come)
We have initial customers, giving us a tight feedback loop, and pays us
We intend to find PMF with our Unique Selling Point at this stage, across either:
Affordable, High throughput support
SOTA Multi-lingual support
Support for enterprise private cloud / on-premise deployment support
We are scaling to GPT 3.5 for Stage 3A, Followed by GPT 4 for Stage 3B
All while building the open-source AI model, open source under the Linux Foundation
And creating that looping wheel, of profits, powering model improvements, in a stable recursive manner.
That makes both investors and employees happily rewarded.
A loop I wish to see repeated across more competing open source models.
If that makes sense to you, feel free to reach out
For investors: founders@recursal.ai
For those who want to join us: hr@recursal.ai
Recursively: Making AI, for everyone, in every language
~ Until next time 🖖 live long and prosper